Everything we do at Discover starts with a focus on the customer. So, when ChatGPT, Microsoft Copilot, and other generative AI technologies were introduced in fall 2023, Discover leadership recognized an opportunity to use these new tools to improve our customer experience.
Chief Information Officer, Jason Strle, gave his engineers a three-fold challenge: "Use generative AI to improve the customer experience while reducing risk."
It's critical to contact customers in their preferred method of communications and at their preferred times, so the team decided to use GenAI to improve this part of the customer experience. This article details an innovative solution that uses GenAI to help customer agents identify when to contact specific customers at the right time and in an appropriate way per the customer's preferences, all while accomplishing the difficult task of keeping costs minimal or even reducing compute costs.
Solution success at-a-glance
The solution that the team produced was wildly successful. A few months after the platform was deployed, the team measured their solution's impact, which included:
- Feature embedding enabled Discover to reduce the time-to-market from 7 hours to 4 minutes
- Parallel model training and dataset handling for 30 million records enabled a decrease in the processing time from 6 days to 3 days and increased the dataset coverage from 20% to 100%
- Decisioning runtime improvements with large language models (LLMs) on a 57,000 record dataset enabled Discover to reduce the sentiment analysis time from 2 hours to 30 minutes
- Reduced cloud spend from 12K per month to 2K for CPU to GPU
"The team did a great job adapting and matching the speed with the risk. When Generative AI is helping in a human-in-the-loop scenario it reduces risk and allows for more speed in the delivery. This can be contrasted to scenarios where a Generative AI solution is autonomously interacting with a customer or otherwise making a business decision. In these cases, there are more risk steps to get to production," says Strle.
So how did we see these incredible results? Read on to learn about the solution.
Classifying customer contact preferences
With thousands of customer calls each day, the need to quickly classify customers, particularly on no-call lists, is imperative for staying compliant with financial regulations and for offering the world-class customer service Discover is known for.
There are numerous preferences that need to be classified. Customers could be on no-contact lists, while others only want to be contacted during specific hours or via certain phone numbers. There are even use cases for customers who are traveling or hospitalized who don’t want to be contacted for certain amounts of time.
The team needed to know if they were contacting customers at the right time, in the appropriate way specified by the customer.
The team created a platform to support the use of the GenAI models and data in a cost-effective manner. The goal of the platform was to give data scientists a streamlined way to access the data sources and tools they needed to build and deploy large-language models.
To give data scientists a faster way to start their analytics work, the team created an easy-to-use interface built on a platform that combined cloud-scale data warehousing with on-demand, scalable, and secure analytics capabilities.
Architecture overview
Discover deployed a generative AI model in production using GPU-powered AWS instances and Nvidia drivers. The model uses Llama-2 models to process transcripts daily to identify no-contact classifications.
The following diagram shows the overarching architecture of the solution.
Managing costs with a precise FinOps model
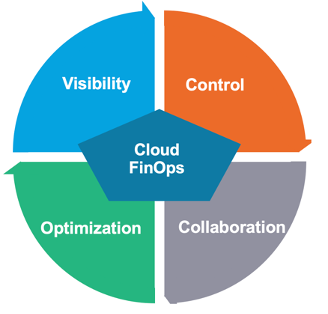
The key with Generative AI technologies is to use them responsibly, both in the way that they use data and also in the way that they use compute resources. While the technology is powerful, it can quickly run up costs because it requires so much resource power to harness large sets of data To that end, the team built cost constraints into the fabric of the platform so that they did not incur excessive costs due to increased CPU usage.
Visibility and transparency were the key to the team’s Financial Operations (FinOps) model. The team set up automated daily checks for cloud users, examining the duration of their server time. Daily emails were sent to managers detailing the costs consumed by their teams and cost dashboards were set up so that the leadership team could review cloud usage. This transparency and visibility worked as a control to ensure the responsible usage of compute resources.
With this visibility, teams could optimize their cloud usage and ensure the GenAI usage wasn’t causing costs to exceed the acceptable thresholds.
Balancing innovation with security
When approaching the use of Generative AI, Discover takes a conservative approach that balances the need for innovation and speed with the need for security and trust with customers.
The first use case of leveraging GenAI to improve the customer service experience for Discover customers has had promising results, and we look forward to seeing other ways we can use the technology.
Learn more
Watch the presentation: Will Hinton and Rahul Gupta presented this project at the AWS Financial Services Symposium.